“Explore how big data and analytics are transforming clinical research efficiency, from patient recruitment to predictive analytics and precision medicine. Learn about the future of data in healthcare.”
The combination of big data and analytics is giving rise to a new efficiency in the design of medical studies, transforming its conduct, and speeding up discovery. This article will explore the implications for clinical research efficiency – including where big data may benefit or challenge us and what to watch out for in the future.
Big Data in Healthcare
Big data is characterized by the high volume of structured and unstructured information that floods healthcare. These include electronic health records (EHRs), genomic data, clinical trial data, medical imaging, sensor or wearable device data and so on.
Efficiency in Clinical Research:
The efficiency of clinical research refers to the optimal conduct of studies using least time, consistent with low cost with maximum benefits. Conventional research methods can be slow to recruit and expensive with restricted data access.
Digital Transformation
The use of digital technologies and large scale data analytics, have together ignited a process of digitisation in the clinical research ecosystem. The transformation includes the procedures of collecting data, analyzing them to derive meaning and share it.
Patient Recruitment:
Big data analytics allows targeted patient recruitment, identifying suitable candidates based on specific criteria like demographics, medical history and genetic predispositions. This allows us to recruit targeted groups faster and also increases diversity in study participation.
Real World Evidence (RWE):
Big data helps in generating real world evidence by analysing data from various sources such as electronic health records (EHRs), claims databases and wearable devices. RWE complements data obtained from traditional clinical trials and assesses the impact of treatment in terms of effectiveness, safety and outcomes in real-world settings.
Predictive analytics:
This type of big data uses patient outcomes, risks, and trial design to predict what will happen. Using historical data and patterns, predictive analytics improve decision-making and reduce uncertainties inherent to trials.
Precision Medicine:
By analyzing patient-specific genetic, clinical, and lifestyle data, big data-driven approaches enable precision medicine. Such a personalized approach takes into account unique traits and thereby administers more effective treatments without adverse events.
Regulatory Compliance:
Big data analytics also plays a role in regulatory compliance and post-market surveillance. Regulators like FDA are now using these methodologies to evaluate the safety and efficacy of drugs. Big Data helps regulatory compliance through holistic evidence and the feature of continuous monitoring.
Patient Privacy and Data Security: Big data is beneficial, but it also raises concerns about patient privacy and security of the data. Strict precautions are required to protect sensitive health information and to comply with regulations, such as HIPAA.
Interoperability: To utilize big data to its fullest, it is important that disparate healthcare systems achieve interoperability among the various platforms. Interoperable platforms, secure data exchange protocols, and standardization of data formats enable seamless integration and analysis of data.
Artificial intelligence (AI): AI algorithms such as machine learning and natural language processing can be incorporated to boost the analytics potential of big data in clinical research. AI-based insights enable optimisation of trial protocols, discovery of new biomarkers and prediction of treatment outcomes.
Furthermore, collaboration and partnerships in academia and among industry partners or government entities are imperative for utilizing a full potential of big data within clinical research. Collaborative partnerships promote information sharing, resource pooling, and joint innovation.
Cost Reduction: The use of big data analytics in clinical trials helps to automate processes which leads to a decrease in expenses related to patient recruitment, monitoring and data management. Big data lowers the financial barrier for research by reducing the waste and streamlining the resources.
Conclusion
This big data integration has set clinical research on a transformative path towards greater efficiency, precision and innovation. With cutting-edge analytics, real-world evidence and collaborative networks at their disposal, stakeholders can speed discovery and better serve patients. With the continual evolution of the healthcare landscape, big data will be critical in determining towards which directions clinical research and medical science progresses.
Reach out to BioBoston Consulting today, or explore the additional ways we can benefit your organization on our website.
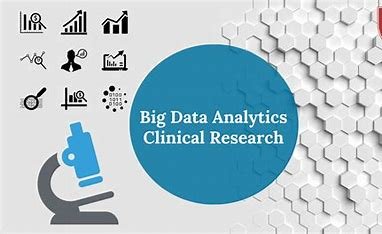